A Scientist is Using A Generative AI Model To Create A Summary Of A Research Paper. The Scientist Then Enters The Prompt Expand More On Point Number 3 And Gets A More Detailed Response. How Is Gen AI Able To Do This ?
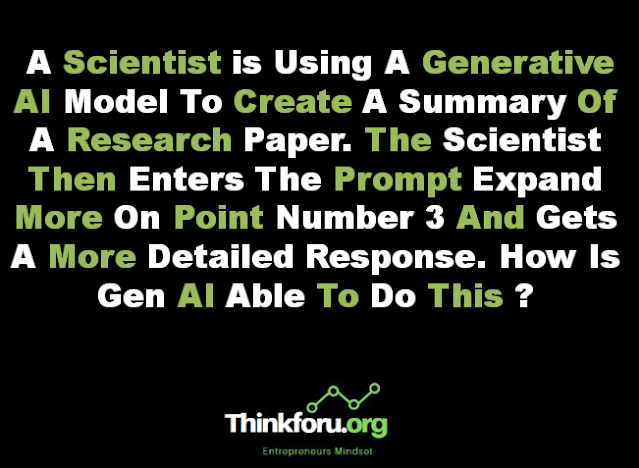 |
Cover Image Of A Scientist is Using A Generative AI Model To Create A Summary Of A Research Paper. The Scientist Then Enters The Prompt Expand More On Point Number 3 And Gets A More Detailed Response. How Is Gen AI Able To Do This ? |
Generative AI models, like GPT-3, are designed to generate human-like text based on the input they receive. These models are trained on diverse datasets containing a wide range of language patterns and structures. Here's how a generative AI model like GPT-3 could generate a more detailed response when asked to expand on point number 3 in a research paper:
1. Understanding Context:
The AI model has been trained on a vast amount of text data, including research papers. It learns the context of various topics and understands the structure of scientific writing.
2. Parsing Prompt:
When the scientist enters the prompt to expand on point number 3, the AI analyzes the provided information and identifies the context related to point 3 in the research paper.
3. Retrieving Relevant Information:
The model retrieves information from its training data that is pertinent to point number 3. It recognizes keywords and phrases associated with that point and gathers relevant details.
4. Contextual Generation:
Using the learned context and information, the AI generates a more detailed response. It understands the style of the research paper and produces text that aligns with the given context.
5. Language Fluency:
The AI model is proficient in generating coherent and contextually relevant language. It can seamlessly extend the information, maintaining the tone and style similar to the original research paper.
6. Adapting to Input:
The model is designed to adapt to different prompts, allowing it to generate diverse responses based on the specific input provided by the scientist. This adaptability enables it to elaborate on specific points as requested.
Let's delve deeper into the mechanisms that enable a generative AI model to provide more detailed responses:
7. Attention Mechanism:
Generative models often use attention mechanisms, allowing them to focus on specific parts of the input sequence when generating the output. In the context of expanding on a specific point, the model can selectively attend to relevant information related to point number 3 in the research paper.
8. Fine-Tuning:
Some generative models, including GPT-3, can be fine-tuned on specific tasks or domains. If the AI has been fine-tuned on scientific literature or research papers, it may have a more targeted understanding of the language and concepts within this domain.
9. Pattern Recognition:
Generative models excel at recognizing and reproducing patterns present in the training data. By identifying patterns associated with the structure of research papers and the language used to elaborate on specific points, the model can effectively generate detailed responses.
10. Incorporating Examples:
The model has encountered numerous examples during training where authors have expanded on certain points in research papers. It can draw upon these examples to simulate a realistic and informative extension of information related to point number 3.
11. Semantic Similarity:
The AI model understands semantic relationships between words and phrases. When prompted to expand on a specific point, it can intelligently choose words and phrases that are semantically related to the context, contributing to the coherence and relevance of the generated content.
12. Probabilistic Output:
Generative AI models output probabilities for the next word or sequence of words. When expanding on a point, the model considers various possibilities and selects the most probable and contextually fitting continuation, contributing to the richness of the response.
In essence, the generative AI model's ability to understand context, retrieve relevant information, adapt to input, and generate coherent and detailed text stems from a combination of sophisticated training methodologies, architecture design, and the vast amount of diverse data it has been exposed to during the training process.
It's important to note that while generative AI models like GPT-3 can generate impressive text, they do not possess true understanding or consciousness. Their responses are based on statistical patterns learned during training and might not always reflect accurate or up-to-date information. Users should carefully review and validate the generated content, especially in scientific or critical contexts.